Video Summarization
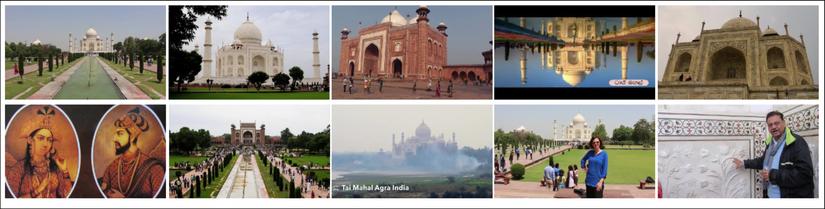
With the recent explosion of big video data over the Internet, it is becoming increasingly important to automatically extract brief yet informative video summaries in order to enable a more efficient and engaging viewing experience. As a result, video summarization, that automates this process, has attracted intense attention in the recent years. Similar to many other areas of video analysis, some of the most effective summarization methods are also learning based. We have explored methods for learning based summarization that requires very weak and limited supervision.
One common assumption of many existing video summarization methods is that videos are independent of each other, and hence the summarization tasks are conducted separately by neglecting relationships that possibly reside across the videos. We investigate how topic-related videos can provide more knowledge and useful clues to extract summaries from a given video. In our CVPR 2017 and TIP 2017 papers, we developed a sparse optimization framework for finding a set of representative and diverse shots that simultaneously capture both important particularities arising in the given video, as well as, generalities identified from the set of topic-related videos. We also showed how web video collections can be effectively summarized with only weak video-level labels [ICCV 2017]. In a subsequent work, we developed a novel multi-view video summarization framework by exploiting the data correlations through an embedding without assuming any prior correspondences/alignment between the multi-view videos, e.g., uncalibrated camera networks.
Within the domain of the summarization problem, but in a different direction, we have analyzed the issue of real-time summarization, which we termed as fast-forwarding through a video. For many applications with limited computation, communication, storage and energy resources, there is an imperative need of computer vision methods that could select an informative subset of the input video for efficient processing at or near real time. In our CVPR 2018 paper, we introduced FastForwardNet (FFNet), a reinforcement learning agent that gets inspiration from video summarization and automatically fast-forwards a video and presents a representative subset of frames to users on the fly. It does not require processing the entire video, but just the portion that is selected by the fast-forward agent, which makes the process very computationally efficient. A distributed version of FFNet was presented in MM 2020.
This work has been supported by NSF and ONR.